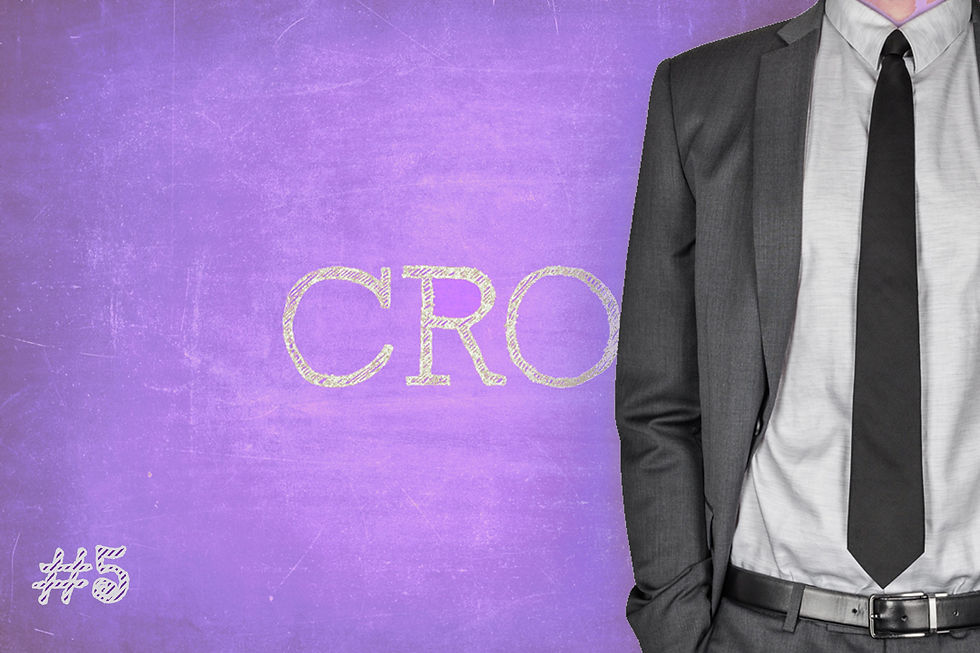
#5 Risk Measures
The risk measures chosen will influence the way risk is seen, interpreted and evaluated in an organisation. This may be either intentional or unintentional; and for sound reasons and occasionally for nefarious reasons. Though surprising, the choice of which risk measures to work with, and which to focus on in a particular instance, is one of judgement and not necessarily a purely analytic choice. It is not an area to neglect as a CRO.
Introduction
What is a risk measure? I will start from first principles and explain how to create and use them and why you should be careful of depending on only one measure.
A useful risk measure should have a set of useful properties - which may seem self evident. First it should increase when ‘risk’ increases. Locally equivalent risk increases should be of the same magnitude as the increase in the measure. A total risk is never greater than the sum of its sub-components.
A risk measure is an attempt to create an algorithm from the data and information you have, and then reduce this to a single dimension (risk as a number, though the dimensional reduction may be to a reduced dimension). There will be many variables and conditionalities on the set of things that determine the change in this number. A risk measure is a projection of all these things into this single number. It can not be perfect in all situations for a number of reasons. Firstly some risk will not be included in the measure – most likely in used examples are from the convexity and large scale properties of the risk. But because risk is a dimension reduction, there will be risks which may be invisible to your metric. (This is like looking at the shadow of a cube on a piece of paper. You can turn the cube until the shadow is a square and you lose information – the 2-d representation of the 3-d shape now is a 2-d shape only). This loss of risk sensitivity is a special dimension (in the sense that it is unlikely to occur in a random portfolio), and may seem not to affect you - except that if you have economic agents looking to reduce risk versus return they may find low (or zero) risks under your measure with inappropriate returns for the risk they carry. This turns out to be a very significant point. It is a selection bias.
In fact this is an example of Goodhart’s law (‘When a measure becomes a target, it ceases to be a good measure.’). Goodhart’s law often plays out in other areas with constructed risk measures – examples include where you form a measure from aggregating KPIs.
A simple way the risk measure may not be sensitive to risks is by construction. If a risk does not feature in a measure then it is not sensitive to it. This basic point has an obvious solution – if your measure is insensitive to a risk then add it to the measure. Yet the risks that are missing from measures are ones missing due to choice (this risk is immaterial relative to others we are measuring, or at least small when you created the measure) or because they are unknown or not visible due to assumptions in the initial construction.
Expected and Unexpected Loss
Most well constructed measures will be based on either sensitivity analysis or probability analysis. Sensitivity analysis are statements such as the change in value of your portfolio with a one basis point (1bp = 0.01%) change in interest rates. Sounds a sensible sort of thing. But how likely are all interest rates in the world at all points of a yield curve to move 1bp? Hence these turn out to have measure issues underlying them. Also the probability of the sensitivity scenario (because it is a scenario, though this may not be how many people think about a risk factor) may be hard to estimate, or not make sense in aggregate across many instruments.
So how do we know the probability of an event? Of course the answer is you don’t know. But an estimate may be available. The simplest available is the historical probability of an event happening being the probability of the event recurring. Which may seem a dumb sort of assumption when it is written down, but is pretty much the underpinning assumption of most aggregate risk measures. These types of assumption generally underpin the probability type risk measures.
An expected loss is in simplest form the amount of loss that is predicted to occur on average. In credit this may be the probability of a default multiplied by the loss on that default. So for a very strong credit this ‘expected loss’ is not very likely to happen at all. (Because mostly no default occurs, but occasionally a default happens and a large loss occurs. Over a large enough portfolio of strong credits – if your measure is right – the sum of the expected loss gives the amount of loss the portfolio may suffer). Anyone who is an expert will now be shouting at the screen… This example is to draw out the fact there are different types of the probability type of risk. There are ones that try to deal in averages over sets of outcomes. Then there are those which try to measure points on the loss distribution, and hence allow for an expected loss you expect to suffer at a low probability. These are VaR-like metrics.
Predicting Expected Losses - VaR
Once we start thinking about VaR or other measures of the tail of a distribution of outcomes, terminology quickly can become fraught with misunderstandings. A VaR is the loss you expect to be exceeded with a set probability. Most VaR models will use historical data from actual days to assess the risk of a large portfolio. To include some risk factors in the calculation, idiosyncratic events may need to be added (say the amount an individual credit spread will vary from the benchmark it is mapped to). The construction will be sensitive to the time periods chosen to measure risk over (10 day VaR versus 1 day VaR), the probability point (99% or 95% are popular), the period of historical data used (2 years, 5 years etc), and whether the historical data are weighted to make more recent events more prominent in the series. Other things that will be important include how missing days/points in data series are filled in. The correlations assumed for these and for any idiosyncratic factors will also be significant.
Sizing Unexpected Losses – Stress
One of the most useful complementary measures to VaR are scenarios and stress measures. Here I take a stress measure to be large moves in a measure – possibly connected to others which will cause losses to a portfolio (say an interest rate and volatility shock). A scenario is a combination of stresses – decided on to be consistent with the world view of the defining context of the scenario (say pandemic or major economic event).
As these are inherently idiosyncratic, it is clear they are prone to miss the move that matters, especially if built by groups that could be conflicted by either good or bad outcomes. Mostly one finds that there is a bit of ‘law of large numbers’ (as popularly imagined) at work, meaning that when organisations suffer large losses they tend to be in the context of scenario scale amounts. This is not coincidence. The scenario loss an organisation is willing to put up with is one of its primary risk appetites. There is a survivor bias in the organisations which back-test these events.
The key in using scenarios is to make sure they seem at least consistent, and where they differ from historical equivalents there is a justifiable reason. An example would be where Italian government bonds go from rallying in some risk off crises in the past to selling off later in the cycle. Going back and testing whether your scenario is stressing your largest concentrations as they vary is necessary on at least a yearly basis.
Forming Measures for Hard to Measure Risks
The main type of modelled risk at question here are operational risk measures. They tend to suffer from extrapolation problems to assess low probability events in models where the model is very sensitive to the assumptions made. Stressing your model for sensitivity to these assumptions is very important as it will give a clear view of the knowability of the real probability or the real magnitude of the event. Most of these models also suffer from bias in that they contain only the things that have happened, not those that were avoided or did not happen. Hence they may deal in mitigated risks rather than unknown unmitigated risks. The models will generally underestimate operational risk by construction.
Sometimes we want to create risk measures for things which are even harder (or problematic) to put monetary value on. For instance a culture metric for a bank. Here the general technique is to use order statistics and assign numbers to them; then sum them in some way. These metrics then to suffer from Goodhart’s law in spades. But they do have some utility, as they give a simple way to combine many factors together for senior audiences, where the detail will generally obfuscate rather that inform.
I hope the above was informative. It is not a scientific paper and hence I have run a bit fast and loose with definitions and terms. If it has sparked interest in making sure you explore the idiosyncrasies of measures which you may take for granted, I will feel I have achieved something.
Next up capital, then liquidity. And hopefully with less delay than this one!
Lewis O'Donald
26th June 2024
Comments